Isabelle Smith
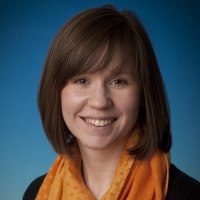
Isabelle is a Principal Statistician based in the Surgical Interventions, Diagnostics and Devices division, at Leeds Institute Clinical Trials Research. In this role she is the statistical lead for wound prevention trials, and surgery trials with interest in orthopaedics, vascular and emergency surgery.
She has recently submitted her thesis as part of an NIHR Doctoral Fellowship investigating the use of multi-state models for the design and analysis of pressure ulcer prevention trials.
Free Paper Presentation at the WReN Scientific Meeting, Glasgow, May 2022
Methods to handle missing data for pressure ulcer prevention clinical trials
Background – During clinical trials, data are collected according to a pre-specified assessment schedule, and common protocols are useful for comparisons between studies. Pressure ulcer (PU) onset and progression in hospital can be modelled as a longitudinal process.
Multi-state models (MSM) are structures that can represent transition of patients through different disease categories (states). MSM can lead to increased power for clinical trials, compared to analysis of aggregated data using binary or time to event methods. However, longitudinal data often suffers from intermittent missing measurements, which may depend on the true disease state. Furthermore, PU outcomes are commonly assessed by collecting PU status of multiple skin sites at multiple time points within a patients, before aggregating to a single binary or time to event endpoint. Ignoring missing data at a skin site level may lead to under-estimation of the true PU incidence which could lead to biased treatment effect estimates.
Methods – The quantity and reason for missing outcome data were explored in a PU prevention trial case study dataset. The potential association with the true (missing) outcome was assessed by a clinical expert, independent of the treatment allocation to inform how the missing data should be handled in the analysis. Candidate definitions for PU outcomes in the presence of missing skin site level data were proposed for our motivating example and the sensitivity of the analysis to these definitions were assessed.
Results – Exploration of the motivating trial dataset identified 5 ‘key’ skin sites that would be associated with the true PU state and would be informative if missing.
Analysing the trial dataset under different definitions of PU outcome demonstrated that the point estimate and precision (and therefore hypothesis tests) of treatments effects were consistent.
The analysis demonstrated that the probability of an outcome being missing was higher for non-healthy outcomes compared to healthy outcomes.
Discussion – Whilst this motivating example demonstrated that the analysis was insensitive to missing data, careful consideration for how to handle missing data across wounds research is required. The reasons for missing data should be collected to help researchers determine the likely association with the true outcome.